AI-Enabled Cybersecurity: A Game Changer in Detecting and Responding to Advanced Persistent Threats
The effectiveness of AI-enabled cybersecurity services in detecting and responding to advanced persistent threats (APTs) is a critical area of focus for organizations aiming to bolster their defenses against sophisticated cyber-attacks.
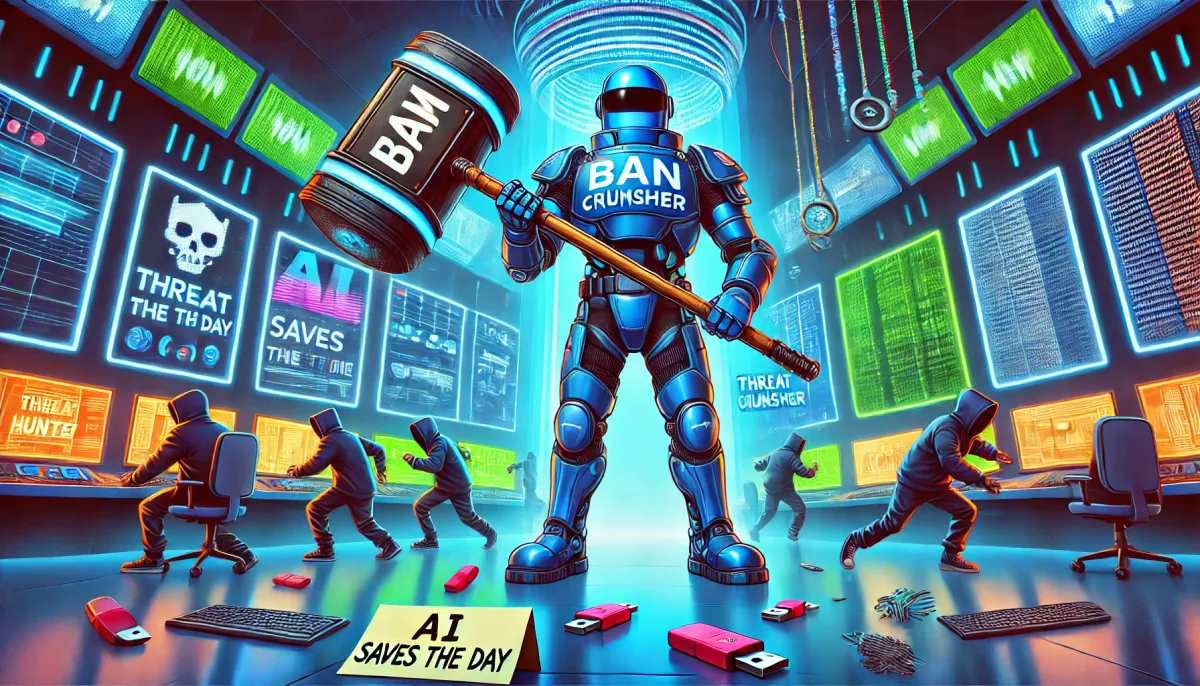
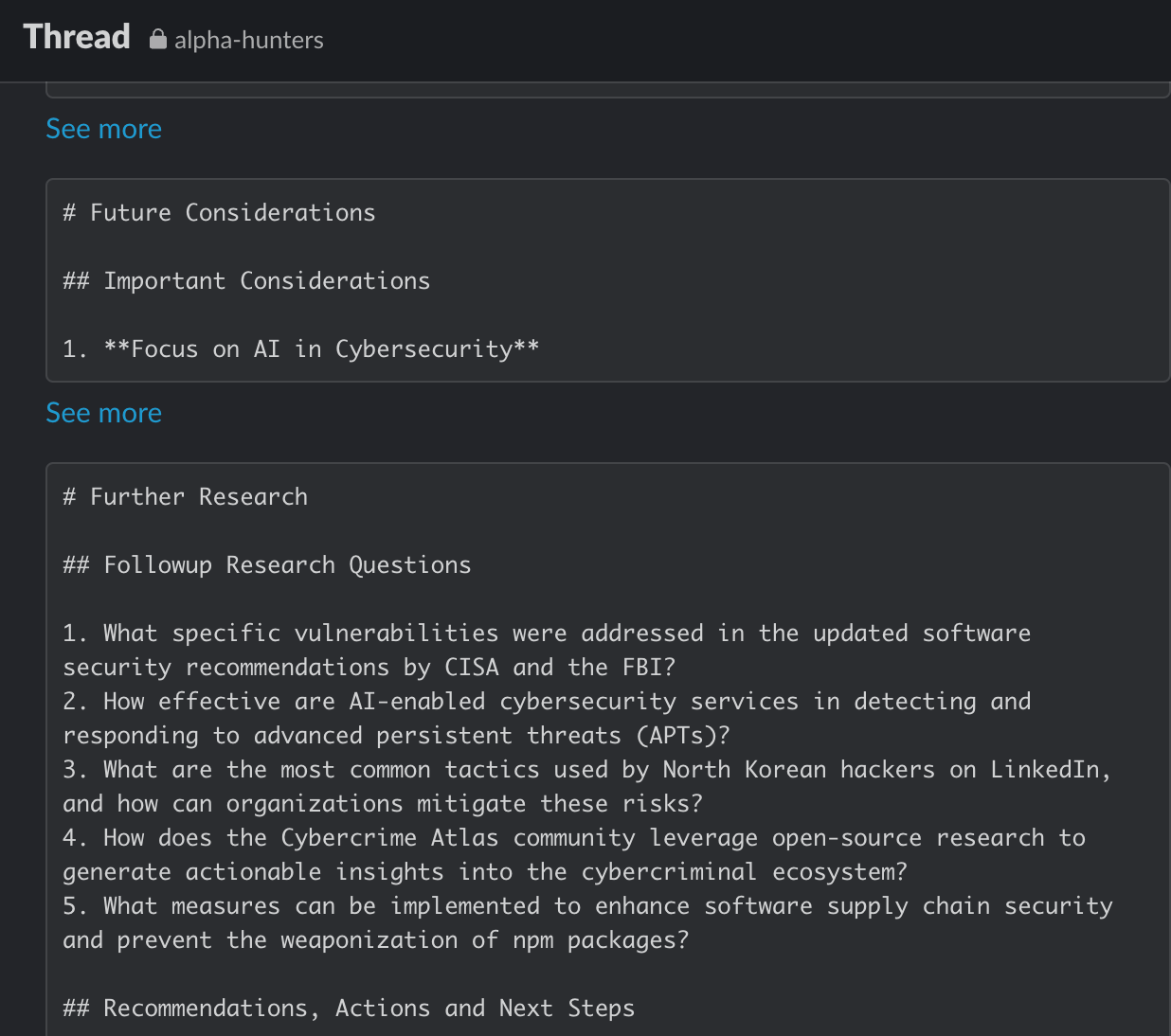
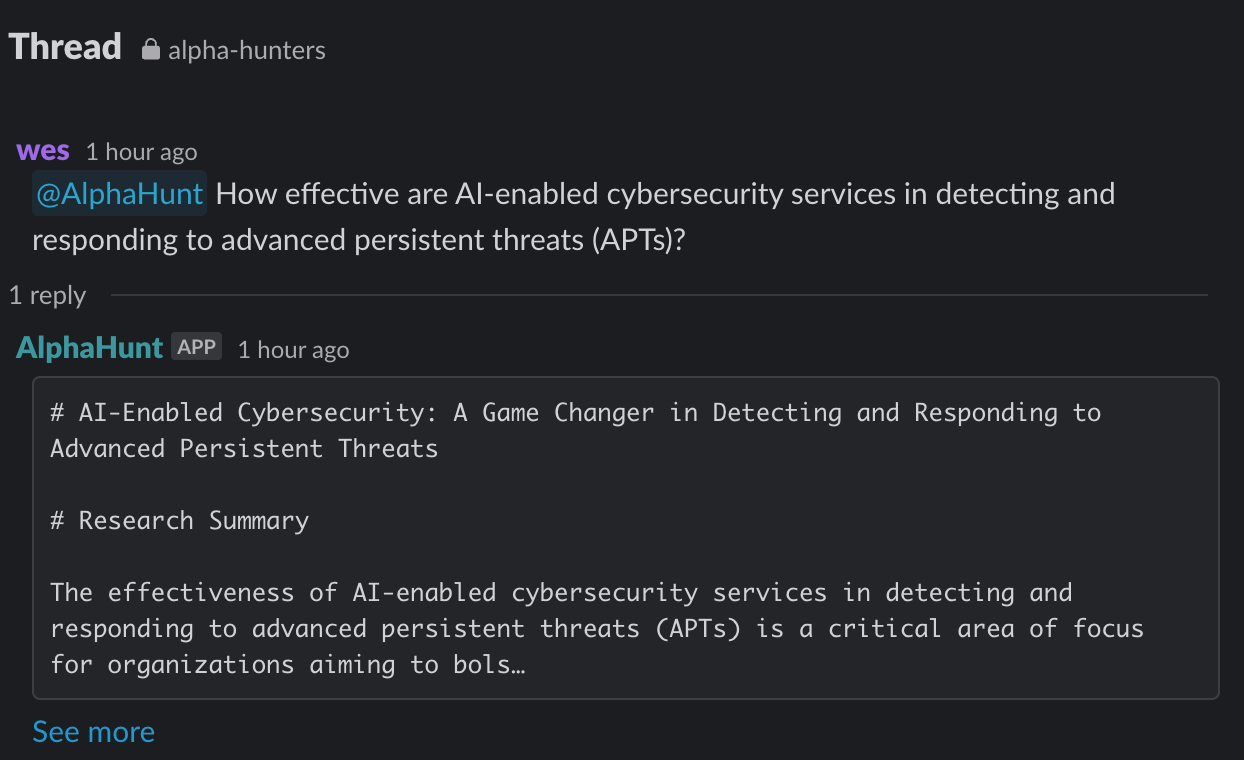
I use this just about every day- easy way to get the contents of a research theme into my intelligence graph for future research.
TL;DR
- Enhanced Detection Accuracy: AI improves detection accuracy by analyzing vast amounts of data.
- Faster Response Times: Automated AI systems can respond to threats in real-time.
- Reduced False Positives: AI models can minimize false positives.
- Scalability: AI systems can scale to handle large volumes of data.
- Integration with Existing Security Infrastructure: AI can be integrated with existing security tools and systems.
Research Summary
The effectiveness of AI-enabled cybersecurity services in detecting and responding to advanced persistent threats (APTs) is a critical area of focus for organizations aiming to bolster their defenses against sophisticated cyber-attacks. APTs are characterized by their prolonged and targeted nature, often causing significant damage to organizations. AI-enabled cybersecurity services leverage advanced techniques such as machine learning (ML) and deep learning (DL) to enhance threat detection and response capabilities, making them indispensable in the modern cybersecurity landscape.
Enhanced Detection Accuracy
AI significantly improves detection accuracy by analyzing vast amounts of data and identifying subtle patterns that may indicate an APT. This capability is crucial for early detection and prevention of sophisticated threats. AI's ability to process and analyze data at scale allows it to detect anomalies that might be missed by traditional methods, providing a more robust defense against APTs.
Faster Response Times
Automated AI systems can respond to threats in real-time, significantly reducing the time between detection and mitigation. This rapid response is essential in minimizing the impact of APTs. AI-driven Security Orchestration, Automation, and Response (SOAR) systems streamline incident response processes, enabling quicker containment and remediation of threats.
Reduced False Positives
AI models can minimize false positives by accurately distinguishing between legitimate activities and potential threats. This reduction in false positives allows security teams to focus on genuine risks, improving overall efficiency and effectiveness. Continuous learning and adaptation of AI models ensure they remain effective against evolving threats.
Scalability and Integration
AI systems can scale to handle large volumes of data and network traffic, making them suitable for organizations of all sizes. Additionally, AI can be integrated with existing security tools and systems, enhancing their capabilities and providing a comprehensive view of potential threats. This integration facilitates improved decision-making and a more cohesive security posture.
Breaches and Case Studies
-
(2024-09-20) Akitra's AI for Advanced Persistent Threat (APT) Detection and Mitigation
- Description: Akitra's AI-driven solutions have been instrumental in detecting and mitigating APTs by leveraging machine learning and deep learning techniques.
- Actionable Takeaways: Implement AI-driven anomaly detection and predictive analytics to enhance threat detection capabilities.
- References: Akitra
-
(2024-10-25) Rackspace's AI Enhancing Threat Detection & Response
- Description: Rackspace's AI-driven threat detection and response systems have successfully identified zero-day exploits and mitigated ransomware and DDoS attacks.
- Actionable Takeaways: Utilize AI for real-time threat detection and automated incident response to improve cybersecurity posture.
- References: FAIR
-
(2024-08-04) Comprehensive Review of AI-driven Detection Techniques
- Description: A review of over sixty studies on AI-driven detection techniques, highlighting the effectiveness of ML and DL in identifying and responding to various cyber threats.
- Actionable Takeaways: Continuously update AI models with new threat intelligence to maintain effectiveness against evolving threats.
- References: Journal of Big Data
Forecast
Short-Term Forecast (3-6 months)
-
Increased Adoption of AI-Driven Threat Detection Systems
- Detailed Analysis: Organizations will increasingly adopt AI-driven threat detection systems to enhance their cybersecurity posture. AI's ability to analyze vast amounts of data in real-time and identify subtle patterns indicative of APTs will drive this trend. For example, platforms like Darktrace's Antigena are already being widely used to detect threats in real-time by learning normal network behavior and identifying anomalies.
- Examples and References:
-
Enhanced Incident Response Capabilities
- Detailed Analysis: AI-enabled cybersecurity services will significantly improve incident response times by automating the analysis of security logs and tracing the point of intrusion. AI-powered SOAR (Security Orchestration, Automation, and Response) systems will become more prevalent, enabling rapid containment and remediation of threats.
- Examples and References:
- (2024-10-23) Cybersecurity Testing in 2024: Impact of AI
- (2024-10-25) Rackspace's AI Enhancing Threat Detection & Response
-
Reduction in False Positives
- Detailed Analysis: AI models will continue to improve in distinguishing between legitimate activities and potential threats, thereby reducing false positives. This will allow security teams to focus on genuine risks and improve overall efficiency.
- Examples and References:
Long-Term Forecast (12-24 months)
-
Integration of AI with Existing Security Infrastructure
- Detailed Analysis: Over the next 12-24 months, AI-enabled cybersecurity services will be increasingly integrated with existing security infrastructure. This integration will enhance the capabilities of traditional security tools and provide a comprehensive view of potential threats. Organizations will benefit from improved decision-making capabilities and enhanced detection of network intrusions.
- Examples and References:
- (2024-08-04) Comprehensive Review of AI-driven Detection Techniques
- (2024-10-25) Rackspace's AI Enhancing Threat Detection & Response
-
Continuous Learning and Adaptation of AI Models
- Detailed Analysis: AI systems will continuously learn and adapt to new threats by integrating up-to-date threat intelligence feeds. This continuous learning will ensure that AI models remain effective against evolving APT tactics and techniques. Organizations will need to invest in maintaining and updating their AI systems to stay ahead of cyber threats.
- Examples and References:
- (2024-10-23) Cybersecurity Testing in 2024: Impact of AI
- (2024-08-04) Comprehensive Review of AI-driven Detection Techniques
Future Considerations
Important Considerations
-
Addressing AI Bias and False Positives
- Detailed Analysis: AI systems must be trained on diverse and comprehensive datasets to minimize bias and reduce false positives. Continuous updates and training with new data are essential to maintain the accuracy and reliability of AI-driven cybersecurity solutions.
- Examples and References:
- (2024-10-23) Cybersecurity Testing in 2024: Impact of AI
-
Mitigating Adversarial Attacks on AI Systems
- Detailed Analysis: As cybercriminals become more sophisticated, they may attempt to exploit weaknesses in AI systems through adversarial attacks. Organizations must implement robust security measures, such as adversarial training, to improve the resilience of AI algorithms against manipulated data.
- Examples and References:
- (2024-10-23) Cybersecurity Testing in 2024: Impact of AI
Less Important Considerations
-
Data Privacy Concerns
- Detailed Analysis: While data privacy is a critical concern, it is less immediate compared to the direct threat of APTs. Organizations should enforce robust data protection policies and ensure compliance with regulations like GDPR and CCPA.
- Examples and References:
- (2024-10-23) Cybersecurity Testing in 2024: Impact of AI
-
Complexity and Skill Gaps
- Detailed Analysis: Implementing and managing AI-driven cybersecurity solutions can be complex and require specialized skills. Organizations should invest in training their cybersecurity teams to effectively utilize AI technologies.
- Examples and References:
- (2024-10-23) Cybersecurity Testing in 2024: Impact of AI
Followup Research
- How can AI-enabled cybersecurity services be further optimized to reduce false negatives in APT detection?
- What are the long-term impacts of integrating AI with existing security infrastructure on overall cybersecurity posture?
- How can organizations address the challenges of data quality and computational demands in AI-driven cybersecurity solutions?
- What are the ethical implications of using AI in cybersecurity, and how can they be mitigated?
- How can AI models be continuously updated to adapt to new and evolving APT tactics?
Recommendations, Actions and Next Steps
- Implement AI-driven Anomaly Detection: Utilize AI models to analyze network traffic and user behavior for early detection of anomalies that may indicate APTs. This will enhance the accuracy and speed of threat detection.
- Leverage Predictive Analytics: Use AI to forecast potential threats based on historical data and trends. This proactive approach will enable organizations to address vulnerabilities before they are exploited.
- Automate Incident Response: Integrate AI-driven automation to streamline response processes, reducing the time required to address threats and minimizing human error.
- Continuous Learning and Updates: Ensure AI systems are continuously learning and adapting to new threats by integrating them with up-to-date threat intelligence feeds.
- Enhance Collaboration between AI and Human Analysts: Combine AI-generated insights with human expertise to improve decision-making and response strategies.
APPENDIX
References and Citations
- (2024-09-20) - Akitra's AI for Advanced Persistent Threat (APT) Detection and Mitigation
- (2024-10-25) - Rackspace's AI Enhancing Threat Detection & Response
- (2024-08-04) - Comprehensive Review of AI-driven Detection Techniques
- (2024-10-23) Cybersecurity Testing in 2024: Impact of AI
Mitre ATTACK TTPs
- T1071.001 - Application Layer Protocol: Web Protocols
- T1059.001 - Command and Scripting Interpreter: PowerShell
- T1078 - Valid Accounts
- T1105 - Ingress Tool Transfer
- T1027 - Obfuscated Files or Information
Mitre ATTACK Mitigations
- M1049 - Antivirus/Antimalware
- M1050 - Exploit Protection
- M1053 - Data Backup
- M1030 - Network Segmentation
- M1026 - Privileged Account Management
AlphaHunt
Get questions like this: How effective are AI-enabled cybersecurity services in detecting and responding to advanced persistent threats (APTs)?
Does it take a chunks out of your day? Would you rather be working on more interesting intelligence tasks? Would you like help with the research?
This baseline report was thoughtfully researched and took 5 minutes.. It's meant to be a rough draft for you to enhance with the unique insights that make you an invaluable analyst.
We just did the initial grunt work..
Are you ready to level up your skillset? Get Started Here!
Did this help you? Forward it to a friend!
(c) 2025 CSIRT Gadgets, LLC
License - CC BY-SA 4.0